Introduction
Risk can often be mitigated if one can correctly assess the factors contributing to risk. Generally, risk assessment and risk mitigation require knowing which factors contribute to risk and assessing them precisely. Snow avalanche risk factors vary in how precisely they may be assessed and how relevant they are (Landrø, Hetland, et al., 2020; Landrø, Pfuhl, et al., 2020). We follow McClung & Scherer (McClung & Schaerer, 2006) and refer to precision as the degree of uncertainty associated with the assessment and measurement of an avalanche risk factor, and to relevance as the importance of a factor in the overall risk assessment. The aleatory uncertainty associated with the avalanche decision-making environment and the complexity in assessing core avalanche risk factors have led to the belief that an analytical, knowledge-based approach is reserved to experts (Atkins & McCammon, 2004; McCammon, 2004). This assumption has never been questioned or adequately discussed within the international avalanche community and in the avalanche education literature. If this assumption turns out to be questionable, it opens new opportunities in avalanche training and decision strategies relevant for amateur recreationalists.
If amateur recreationalists identify the same factors as relevant as experts do, then avalanche education can focus on how to assess these factors. If recreationalists think they can assess most of the factors precisely but judge relevant factors as irrelevant, teaching should focus on explaining the relevance of these factors. If recreationalists neither know how to assess the factors nor its relevance, avalanche education must teach the how and why to ensure better decision-making and safe skiing. Factors that are predictive of avalanche risk and at the same time possible to assess with sufficient precision, should be used in decision tools and taught in avalanche courses.
Avalanche risk assessment
An avalanche release depends on the interaction between four main factors: terrain, weather, snowpack, and a trigger (McClung & Schaerer, 2006). These four main factors can be divided into a range of underlying factors (Landrø, Pfuhl, et al., 2020) varying in their relevance and the precision with which they can be assessed. In terrain around 30 degrees inclination and steeper, avalanches can be triggered. Once released, an avalanche can run into much gentler terrain, the runout zone. The release areas and runout zones are referred to as avalanche terrain. Avalanche terrain is a relevant factor, but in the extreme case of not being able to recognize avalanche terrain, knowing that this is a relevant factor for avalanches does not help in judging avalanche risk.
The trigger is either something that weakens the snowpack (i.e., increasing temperature) or something that exerts an additional load on the snowpack (i.e., precipitation) or a combination of the two. Often the trigger is a backcountry recreationalist who adds load that exceeds the strength of the snowpack (Harvey et al., 2018; McClung & Schaerer, 2006; Schweizer & Lütschg, 2000).
A stable snowpack can support a high additional load (e.g., a group of skiers or a snowmobile), whereas a poor stability snowpack may avalanche naturally or with a small additional load (e.g., a single skier). Therefore, slope angle and snow stability are key factors in avalanche risk assessment. Natural processes, such as precipitation, radiation, wind, and differences in temperature, contribute to variations of snowpack properties and its stability. For example, every snowfall increases the avalanche danger, but how much it increases depends on the precipitation intensity, temperature, wind speed, and the composition of the old snowpack. In favorable conditions, when the old snowpack is stable, the temperature at the beginning of the snowfall is mild (about 0oC), and calm winds, 30–50 cm of new snow indicate a critical situation (minimum danger level 3-considerable). In contrast, 10–20 cm of new snow is regarded as critical in unfavorable conditions, typically strong winds, low temperatures at the beginning of snowfall, and a generally weak old snowpack. However, since there are many possible combinations of factors that should or could be assessed, there will always be some residual uncertainty associated with stability assessments (Harvey, 2018).
Knowledge-based approach
Experts do the risk assessments based on knowledge and experience. But what about amateur recreationalists? The traditional view has been that most amateur recreationalists have too little training and spend too little time in avalanche terrain to develop a reliable knowledge-based approach (McCammon, 2004). A knowledge-based approach requires knowledge to see how different factors interact, as well as insight into the limitations of this knowledge, i.e., focused information seeking, cue monitoring, and responding to changes. Rapid decisions can be made if the conditions are deemed easy, e.g., gentle terrain, low danger level, easy recognizable and manageable avalanche problem. In complex situations, the risk assessment requires detailed observations and careful weighting of the risk factors and the uncertainty (Harvey et al., 2018; McClung & Schaerer, 2006; Reuter et al., 2021). The decision-making process becomes slow and typically follows a given structure to support and systematize the process. The Systematic Snow-Cover Diagnosis (Kronthaler, 2019) provides such a structure.
Experiential learning
A knowledge-based approach presupposes insight into relevant factors and an understanding of their interaction. It requires practical skills and exposure to many varied situations in the learning process. Unlike other learning theories, such as cognitive and behavioral learning theories, experiential learning emphasizes experience in the learning process (Kolb, 1984). Experiential learning builds on experience, perception, cognition, and behavior. The latter is of great importance since the use of the acquired knowledge and skills culminates in a decision to expose oneself to potential risk or not. Although this form of learning is probably the most suitable in this context, there are several pitfalls to consider. Efficient learning requires reliable feedback (Stewart et al., 2012). What complicates decision-making in avalanche terrain is limited or absent feedback (Hogarth et al., 2015), making it difficult to develop relevant skills through experiential learning (Elwin, 2013; Ericsson et al., 1993). Backcountry recreationalists might therefore develop a false sense of confidence in their risk management and assessment skills because yet so often wrong or flawed decisions provide positive feedback: no avalanche triggered (Bonini et al., 2019; Stewart et al., 2012). Further, if one considers avalanches as random events, one may not see any benefit in learning about the factors relevant for assessing avalanche risk (Dunlap & Stephens, 2016; Pfuhl et al., 2011; Stephens, 1991). Still, many critical factors and relations could be learned by amateurs as well as experts, and there are ways to accelerate the learning process thereby facilitating knowledge-based approaches for different user groups.
Avalanche factors tested in this study
A previous study identified 53 factors that either are part of a decision-making framework (DMF) or used in expert decision-making (Landrø, Hetland, et al., 2020). We selected 11 of these factors, which emerged as highly relevant among experts or where well-known factors.
The factors inclination and danger level are essential in many DMFs. Inclination is of importance for fracture initiation, fracture propagation, and sliding – processes relevant for dry slab avalanche release. Without an inclination above 30 degrees, avalanches will usually not occur. The danger level (1 – low to 5 – very high) is derived from a set of definitions and is the most prominent part of a public avalanche forecast (Engeset et al., 2018). The factors avalanche terrain (release area and runout zone) and non-avalanche terrain and expected avalanche size (1 – small to 5 – extremely large) are absent in the commonly used DMFs, but are instrumental to experts (Landrø, Hetland, et al., 2020). Stopping at a safe spot is one of several standard travel measures and group management techniques. Its sole purpose is to reduce overall risk. Signs of instability, such as recent avalanches or collapsing whumpfs, are regarded as direct evidence of snow instability and avalanche danger (McClung & Schaerer, 2006). When present, this is easily accessible information, and there should be little uncertainty associated with its interpretation. In contrast, weak layer distance from surface, test score from stability tests, and distribution of weak layer (in which aspects, elevation bands, and terrain formations the layer can be found) are associated with considerable uncertainty in their interpretation. Appropriate use and understanding the limitations of these factors require knowledge and practical experience. To illustrate, take a weak layer, which is a precondition for fracture initiation and propagation, and therefore a relevant factor. One can measure the distance between the weak layer and surface with great precision in one location. However, the distance can vary due to wind distributing the overlying snow unevenly in the terrain, affecting the precision with which this factor can be assessed. Terrain traps are obstacles or terrain features in the avalanche path that increase the consequences in case of an avalanche release. Forest density is a twofold factor, increasing consequence and effecting snow stability. Interpretation of the latter is associated with uncertainty and requires knowledge and practical experience.
Purpose and objectives
Erroneous judgement of avalanche risk may lead to fatal outcomes; thus, the choice of judgement method matters. The purpose of this study was to question the assumption that analytical knowledge-based approaches to avalanche decision-making are applicable to experts only. If this assumption is invalid, there will be new opportunities, and challenges, in both avalanche education and development of assessment methods.
Our first objective was to examine the assessment and judgment of a selection of avalanche risk factors and the role of avalanche education in bridging the gap between amateur and expert recreationalists. Our second objective was to investigate if experience with avalanche incidents or accidents influenced the judgment of relevance of the risk factors, and if the judgement of avalanche risk improves with more knowledge.
Methods and materials
Participants
Participants were panelists and attendees at three avalanche seminars organized by the Norwegian Avalanche Warning Service (NAWS) at the Norwegian Water Resources and Energy Directorate (NVE) and the Center for Avalanche Research and Education (CARE) at UiT – The Arctic University of Norway. The survey language was in Norwegian. Nine percent had Norwegian as second language, but deliberate reasoning is similar in native and second language (Maekelae & Pfuhl, 2019).
Survey
We selected 11 factors and asked how precisely (1 = not very precisely, 2 = fairly precisely, 3 = very precisely) they can assess the factor and how relevant (1 = insignificant, 2 = relevant, 3 = decisive) the factor is for risk assessment. I don’t know this factor was also possible to choose. We counterbalanced whether participants first rated precision and secondly relevance, or vice versa. The order of presenting the 11 factors was constant per test session. With four questions we investigated whether avalanches are regarded as random or predictable (Ulkumen et al., 2016). Two items assessed aleatory uncertainty (McDonalds ω = .638), and two items epistemic uncertainty (McDonalds ω = .539).
We asked about 1) their level of avalanche education (range: 1 = beginner to 7 = expert level); 2) skiing skills and exposure to backcountry skiing (range: 1 = beginner to 7 = expert skills); 3) avalanche accident involvement; and 4) demographic questions, i.e., age, gender, years of backcountry skiing, and average number of backcountry trips during the last three seasons. Avalanche education was a subjective rating along a 7-point scale, though most participants were previously provided with at least two anchor points; beginners had no skills in how to evaluate avalanche danger and experts had profound knowledge of snow metamorphosis and avalanche dynamics. In a follow-up survey among 99 naïve participants the rating on this simple scale correlated .68 with the rating along a scale providing avalanche course descriptions (e.g., 1-day course, 3-day course, NVE 4a, IFMGA) and ordering them into six categories plus a 0th level for no avalanche education.
Procedure
Attendees at avalanche seminars were presented the survey in-between talks related to decision-making in avalanche terrain, where the factors were visually displayed on a large screen (Figure 1) and answering was done on mobile phones. Panelists were contacted via email. The survey was implemented in Qualtrics where participants gave their informed consent.
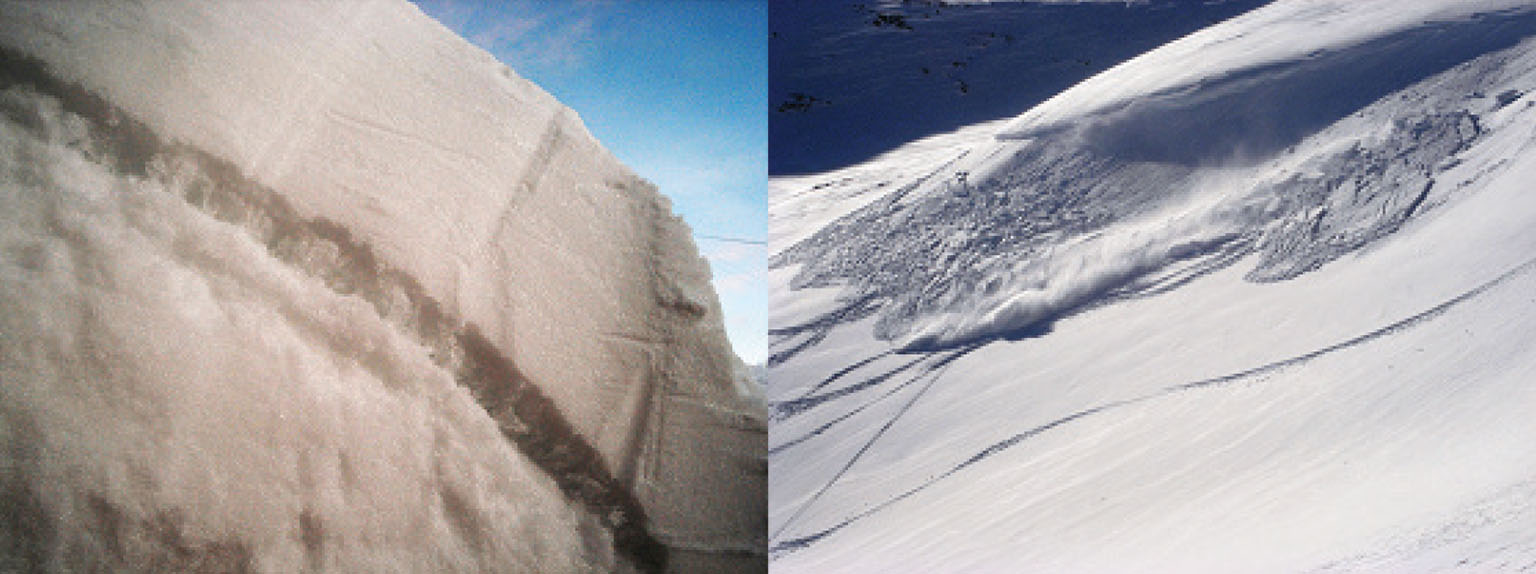
Analysis
We analyzed each factor separately with regards to rating of precision and relevance, and with avalanche education and avalanche accidents as predictors. To gauge the relationship between the rating of the factors and participants’ avalanche education and accidents, we performed a network analysis. To assess whether amateur recreationalists and experts judge relevance similarly, we performed Bayesian Association tests with Poisson sampling (Morey et al., 2018).
The answer options regarding avalanche accident and incident experiences were transformed into an ordinal scale where 0 = “No, I have not been involved in an avalanche incident or accident”, 1 = “I have seen an avalanche been triggered”; 2 = “Someone I was traveling with triggered an avalanche” or “I triggered an avalanche”; 3 = “Someone I was traveling with got caught, but not buried” or “I got caught, but not buried”; 4 = “Someone I was traveling with was buried” or “I have been buried in an avalanche”; 5 = “Someone I was traveling with got injured”; “I got injured”; “Someone I was traveling with died in an avalanche”. If a person selected more than one option, the highest rank was used. Regarding avalanche education we merged the two highest levels, as only very few indicated avalanche education at level 6 and 7, i.e., yielding six levels. To measure whether participants consider avalanches as random or predictable, we averaged the ratings for the two aleatory and two epistemic items, respectively and applied ANCOVAs with avalanche education and accidents as predictors and the rating score on the uncertainty scales as within-factor. Descriptive data is rounded to the nearest percentage (may not add up to 100%). Since we analyze 11 factors, we use a stricter significance level of p < .002, and generally consider both p-value and effect sizes.
Open science
Our pre-registered analysis plan, all material and data can be found at: https://osf.io/k5gzu/
Ethics
Participation was voluntary and anonymous. The study was approved by the National data security agency, NSD number 233888.
Results
Demographics
1,220 participants (64% male) completed at least 80% of the survey (580 at avalanche seminars). The online panelists, on average, were more experienced, both in skiing and with avalanche incidents, they also had more avalanche education (Table 1). 45% had not experienced any avalanche incident, while 3% experienced a severe incident. Most respondents had only basic avalanche education and were rarely involved in severe accidents (Figure 2). Skiing skills, average number of trips, avalanche accidents, and avalanche training level were all positively correlated (.386 > r > .492). The more people were backcountry skiing, the more avalanche training they had, and the more likely they were to have experienced an incident or accident. Overall, our sample is a representative snapshot of the amateur backcountry community.
Avalanche seminars | Online panel | N | Test statistic | ||
---|---|---|---|---|---|
Age: mean (SD) | 36.65 (12.22) | 38.13 (11.54) | 1204 | t = 2.183 | p = .029, d = .126 |
Gender (male/female/other) | 325 / 251 / 4 | 451 / 189 / 0 | 1213 | χ2 = 30.318 | p < .001 |
Ski skills: mean (SD) | 4.03 (1.31) | 4.69 (1.15) | 1219 | t = 9.320 | p < .001, d = .535 |
Average number trips (SD) | 19.25 (20.21) | 22.59 (20.69) | 1218 | t = 2.849 | p = .004, d = .164 |
Avalanche experience (SD) | 1.02 (1.32) | 1.37 (1.4) | 1212 | t = 4.437 | p < .001, d = .256 |
Avalanche education (SD) | 3.01 (1.37) | 3.61 (1.18) | 1220 | t = 8.135 | p < .001, d = .466 |
Legend: SD … Standard deviation.
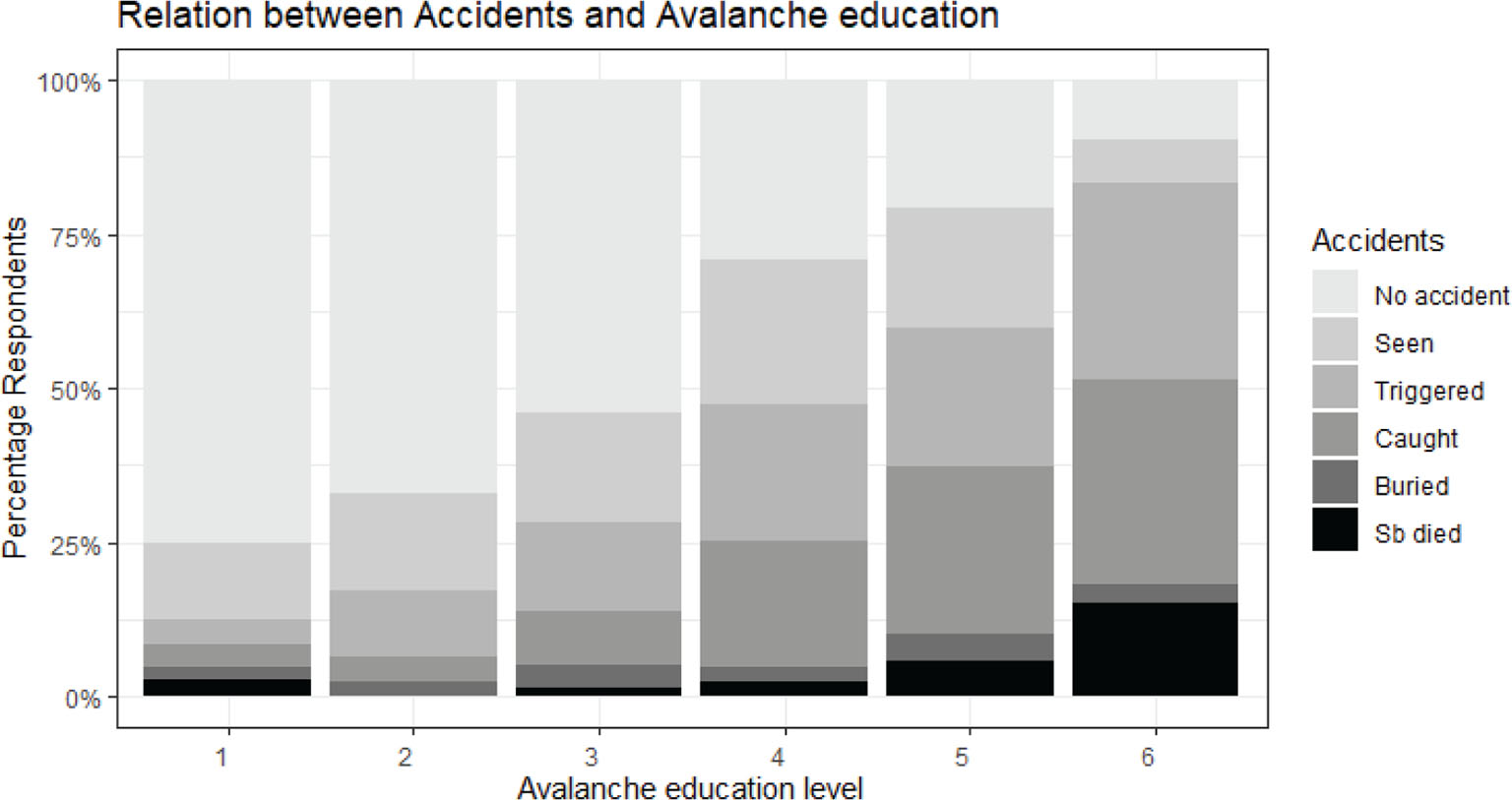
Avalanches are considered predictable
On a scale from 1 to 7, participants’ rating for avalanches being a random event was low; M = 3.01, SD = 1.23; whereas the rating for avalanches being predictable was high; M = 5.41, SD = 1.01. There was a main effect for aleatory vs epistemic uncertainty (F1, 938 = 187.28, p < .001, η2 = .108). There was no main effect of avalanche education (F < 1), but of avalanche accidents (F1, 938 = 5.2, p = .023, η2 = .006). The more severe accidents the less avalanches are seen as random. Epistemic uncertainty rating was not affected by accidents. The interaction between avalanche education and uncertainty (F < 1), and accidents and uncertainty (F < 1) was not significant.
Avalanche education influences precision rating of avalanche factors
The more avalanche education and training the better a factor could be assessed and interpreted. Exceptions were forest density and stability tests, where avalanche education did neither affect the ability to assess the factor nor its relevance. Experience with accidents played a minor role in assessing and judging the factors. Figure 3 shows the results for each of the 11 factors precision and relevance judgements by avalanche education, and Table 2 the regression results.
Danger level was rated as not very precisely by 24%, as fairly precisely by 69% and as very precisely by 7%. Relevance was rated as insignificant by 5%, relevant by 54% and decisive by 40%. Danger level was judged less relevant and less precise to assess the more avalanche education and more severe avalanche experience a person had.
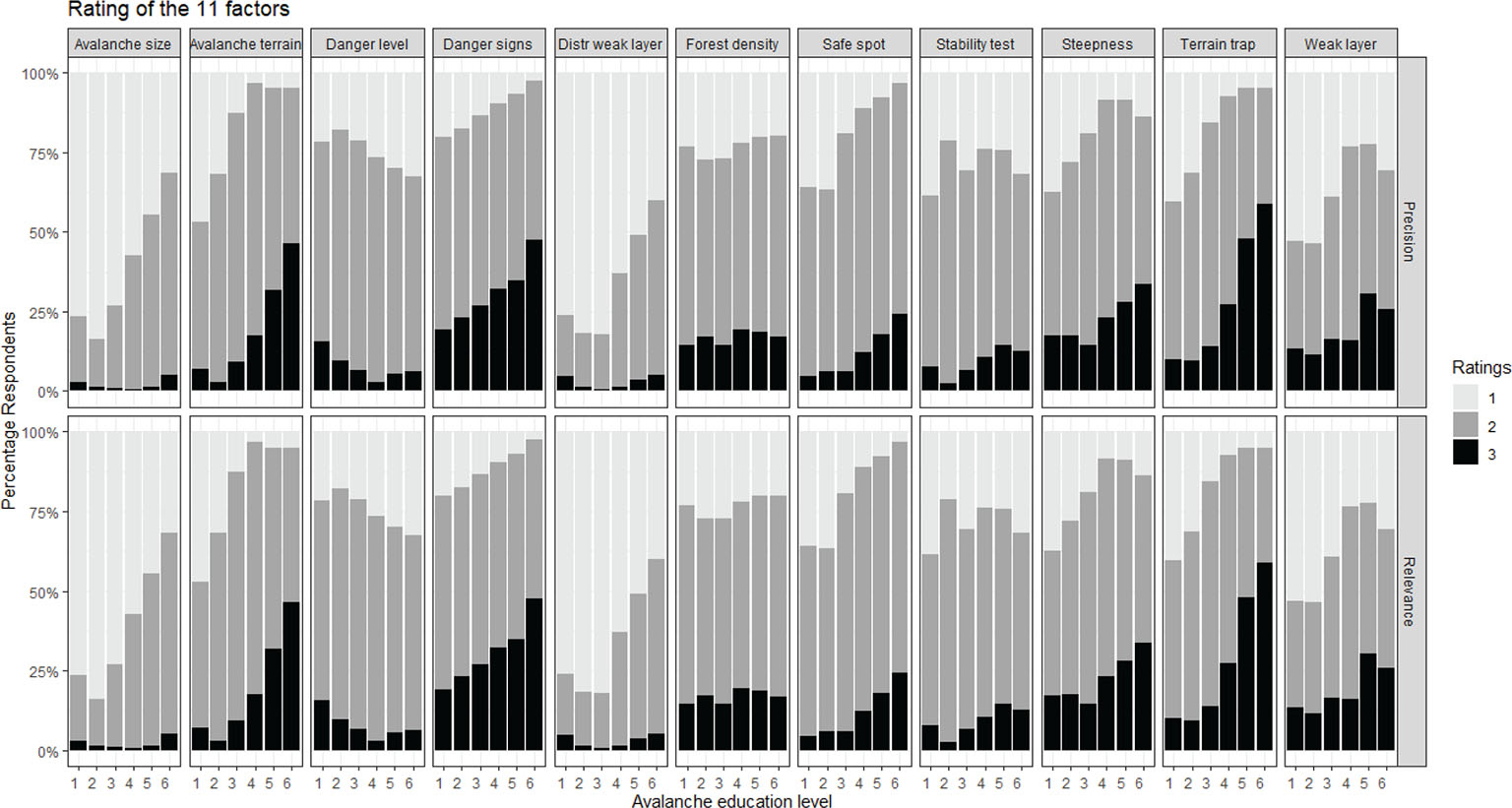
Legend: Top row: Precision rating was: 1 = not very precisely, 2 = fairly precisely, 3 = very precisely. Bottom row: Relevance rating was: 1 = insignificant, 2 = relevant, 3 = decisive. For steepness/inclination the answer option “only care if it is about 30o steep” was excluded (n=170). Danger signs are also referred to as signs of instability.
Avalanche size was rated by 65% as not very precisely, by 33% as fairly precisely, and by 1% as precisely. Relevance was rated by 3% as insignificant, 53% as relevant and 44% as decisive. Assessment of avalanche size improved with avalanche education and experience.
Signs of instability were rated as not very precisely by 12%, as fairly precisely by 59% and as very precisely by 29%. Relevance was rated as relevant by 17% and decisive by 83%. Assessment of signs of instability improved with avalanche education, and was judged as decisive by the majority, irrespective of avalanche education.
Distribution of the weak layer was rated as not very precisely by 70%, as fairly precisely by 28% and as very precisely by 2%. Relevance was rated as insignificant by 2%, relevant by 45% and decisive by 52%. Precision and relevance rating of the distribution of the weak layer improved with avalanche education.
Weak layer distance from snow surface was rated as not very precisely by 36%, as fairly precisely by 46% and as very precisely by 18%. Relevance was rated as insignificant by 5%, relevant by 62% and decisive by 33%. Assessing the weak layer distance from snow surface improved with avalanche education.
56% did not know what a stability test score is. Among those who knew, the factor was rated as not very precisely by 27%, as fairly precisely by 63% and as very precisely by 10%. Relevance was rated as insignificant by 12%, relevant by 63% and decisive by 24%. Avalanche education did not impact the rating of precision or relevance.
Stopping at a safe spot was rated as not very precisely by 19%, as fairly precisely by 71% and as very precisely by 10%. Relevance was rated as insignificant by 4%, relevant by 51% and decisive by 45%. Avalanche education improved the assessment.
Inclination was rated as not very precisely by 18%, as fairly precisely by 61% and as very precisely by 20%. Relevance was rated as insignificant by 1%, relevant by 38% and decisive by 39%. 22% stated that only knowing whether it is above or below 30 degrees matters for them. Assessing inclination improved with avalanche education.
Avalanche terrain was rated as not very precisely by 15%, as fairly precisely by 70% and as very precisely by 15%. Relevance was rated as relevant by 24% and decisive by 76%. Assessment of avalanche terrain improved considerably with avalanche education, whereas relevance was very high irrespective of education.
Forest density was rated as not very precisely by 24%, as fairly precisely by 59% and as very precisely by 17%. Relevance was rated as insignificant by 19%, relevant by 72% and decisive by 9%. Assessment and relevance of forest density was not affected by avalanche education.
Terrain traps were rated as not very precisely by 16%, as fairly precisely by 60% and as very precisely by 23%. Relevance was rated as insignificant by 1%, relevant by 43% and decisive by 56%. Assessment of terrain traps but not relevance improved with avalanche education.
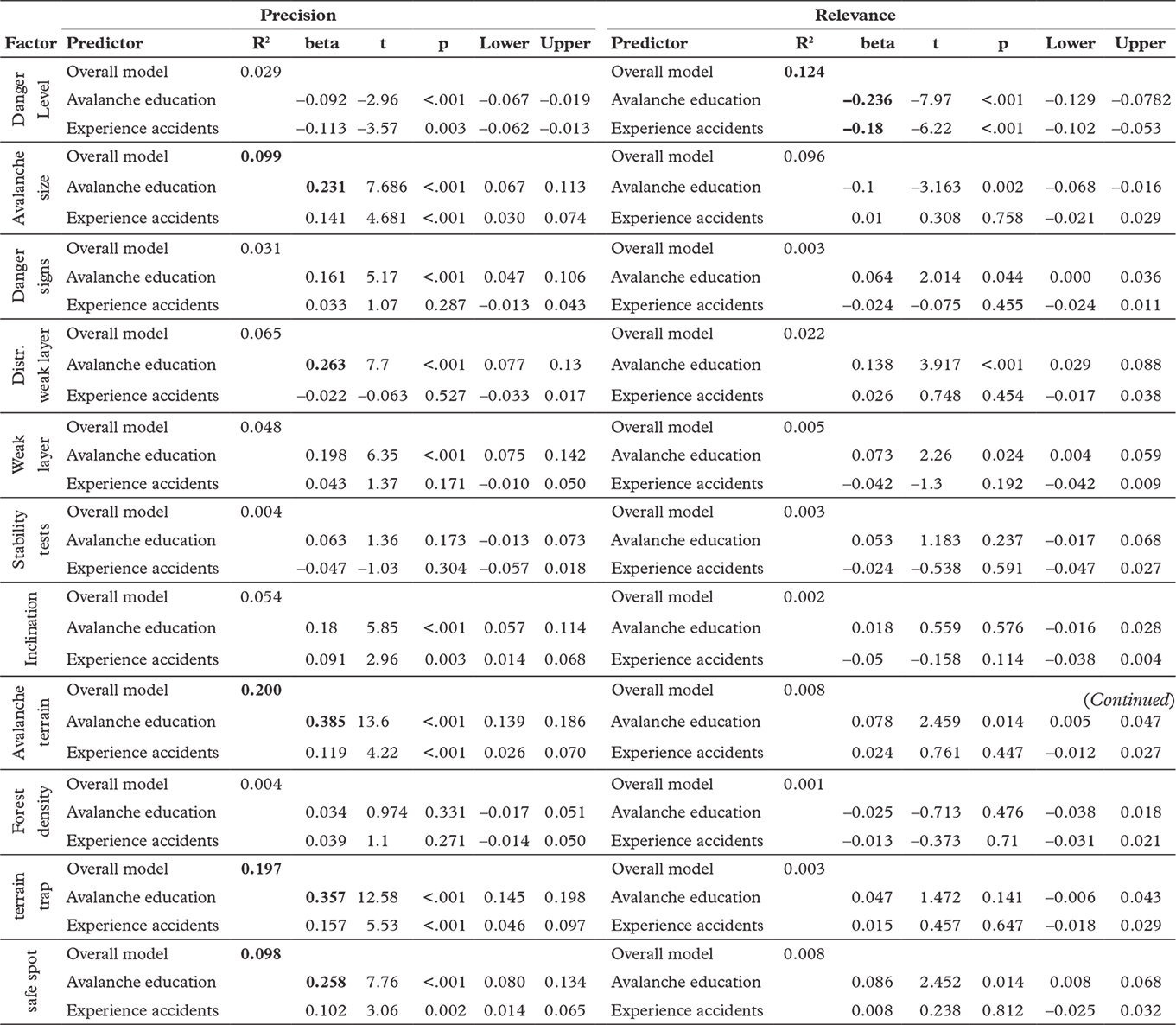
Legend: Lower and upper refer to the 95% Confidence interval of the unstandardized estimate (not reported). Beta is the standardized estimate (effect size) and a beta of e.g., .3 means that with each higher level in education the precision increases by .3 on a scale from 1 to 3, for example, for avalanche terrain with beta = .385 and six levels of education, the difference between education level 1 and 6 yields a change from rating precision at 1 (not very precise) to 3 (very precise). R2 is the explained variance and can be interpreted similar to a Pearson correlation coefficient. 10% explained variance can be in the long run a very meaningful effect (“potentially more ultimately consequential”)(Funder & Ozer, 2019). The first 237 participants received only eight not 11 factors.
Effect of avalanche education and accidents across all factors
We performed a network analysis using weighted least squares estimation for ordered data (polychoric correlations), to assess whether a participant’s rating across the factors as well as their avalanche education and experience with accidents are related. The factor stability test was excluded. A network analysis is a statistical tool to describe the interrelationship of a set of variables, where the nodes represent observed variables that are connected by edges representing their statistical relationships (partial correlations). Here, the variables are the 10 factors, avalanche education and accidents. Figure 4 shows that the precision ratings of all factors but danger level were highly correlated (blue edges) with each other, with avalanche education and avalanche experience (accidents). A negative relationship (red edges) is found for danger level with avalanche education and accidents. Rating of forest density and danger signs are less strongly related to the other seven factors. Regarding relevance judgements, the network is less dense, meaning a participant’s relevance rating of one factor was less related to their rating of another factor. Avalanche education is only weakly correlated with a factor’s relevance rating. The exception is danger level, which is strongly anti-correlated with avalanche training and accidents. Experience of avalanche accidents play no role for relevance ratings, exception is danger level.
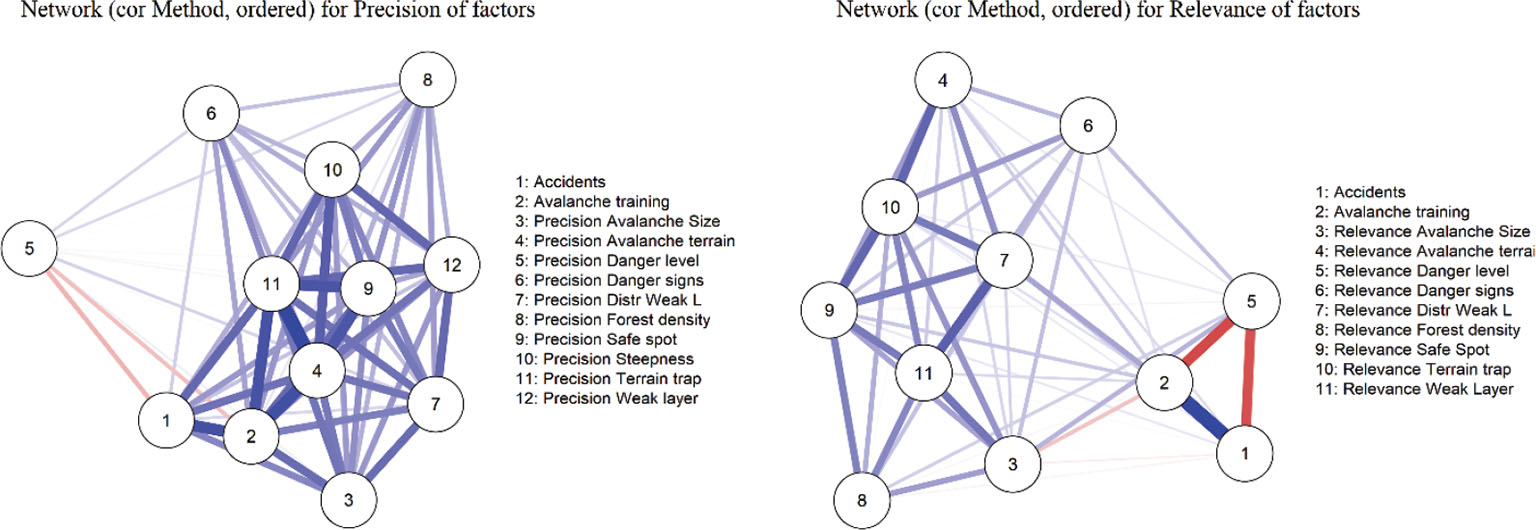
Legend: Left-hand side shows the network for the rating of the precision of the factors, right-hand side for the rating of relevance. Blue lines denote positive relationships, red lines denote negative relationships. The thicker the line, the stronger the relation. Centrality measures can be found on the OSF page of the article.
Comparison to experts’ relevance rating
We applied a test of association to assess how well the relevance ratings made by amateur recreationalists aligns with that of avalanche experts, using results from a previous study on expert’s relevance rating (Landrø et al., 2020b). We report Bayes factor, i.e., the ratio of likelihoods to measure the strength of evidence in favor of parity, here between amateur recreationalists and experts. The Bayes factor was interpreted according to Wetzels and Wagenmaker (2012). The threshold for evidence in favor of no difference was BF01 ≥ 3.0. There was large support for parity for all but the stability test score, i.e., experts and recreationalists rated the relevance of the factors similarly (Table 3).
Factor | Test of association (Bayes factor) | Interpretation |
---|---|---|
Danger level | 71:1 | No difference between experts and recreationalists |
Avalanche size | 286:1 | No difference between experts and recreationalists |
Signs of instability | 49:1 | No difference between experts and recreationalists |
Distribution weak layer | 106:1 | No difference between experts and recreationalists |
Weak layer distance from snow surface | 25:1 | No difference between experts and recreationalists |
Stability test score | 0.5:1 | Neither supporting nor rejecting parity |
Stopping at safe spot | 5:1 | No difference between experts and recreationalists |
Inclination | 770:1 | No difference between experts and recreationalists |
Avalanche terrain | 370:1 | No difference between experts and recreationalists |
Forest density | 48:1 | No difference between experts and recreationalists |
Terrain traps | 11:1 | No difference between experts and recreationalists |
Discussion
In this study, we asked 1,220 amateur backcountry recreationalists about the degree of precision with which an avalanche factor can be assessed and how relevant the factor is for their risk assessment. We compared these results with results from a previous study on expert judgments (Landrø, Hetland, et al., 2020) and found that amateur recreationalist judged the relevance of the tested avalanche factors very similar to experts. For all but danger level, experience with avalanche incidents or accidents did not influence the relevance judgement. Danger level was also unique, as assessing and judging this factor was not related to the rating of any of the other factors. The precision rating depended on avalanche education for most of the factors, and for some factors also on experience with avalanche accidents. We discuss the findings for each of the factors before we discuss some broader implications for avalanche education and forecasting.
Understanding of avalanche factors
The factor danger level, expressed as a number between 1 – low and 5 – very high, is not physically observable, and is obtained by reading an avalanche forecast or assessed in the field. Most of our participants judged the danger level as a factor that is low in precision. The more avalanche education and accidents, the less precise and relevant the factor was rated. Surprisingly, danger level is currently not that well defined by the avalanche warnings services. In fact, the European Avalanche Warning Services (EAWS) are working on (1) the parameters required to define avalanche danger and (2) improving the algorithm needed to derive the avalanche danger from the defining properties (EAWS, 2019). In North America, the defining parameters have been identified and explained, but the algorithm has not been defined (Statham et al., 2018). For recreationalists without any formal avalanche education, the danger level is a relevant factor, but with more training and education the factor becomes less relevant for the risk assessment.
Amateur recreationalists judged expected avalanche size as a relevant factor but considered it difficult to estimate. Expected avalanche size is determined by several factors, i.e., slab stiffness, weak layer properties, destructive potential, length, area, volume, mass, or how far it runs into flatter terrain. All these properties are hard to assess in the terrain and may contradict each other when trying to assign a size category. Estimating avalanche sizes requires training and experience, and indeed the more training a recreationalist had, the higher was the precision rating. The factor’s relevance decreased with more avalanche education. This might be due to more knowledgeable recreationalists distinguishing between avalanches that are large enough to be fatal and those that are small enough that they can be skied out. Still, size matters in terms of how large a runout area one must consider.
There was a large agreement regarding signs of instability’s high relevance. The ability to detect the signs and understand what causes them increased with avalanche education. Recreationalist with no formal avalanche education judged danger signs as moderately precise to assess. No other factor received such a high precision score.
Distribution of weak layer scored high on relevance, and relevance and precision increased with avalanche education. This factor received the lowest score among the 11 factors for precision, and is generally associated with uncertainty in how to interpret it (McClung & Schaerer, 2006).
Weak layer distance from surface was neither rated as precisely to assess nor as decisive, but avalanche training did improve the ability to assess the factor. This might be due to an increased understanding of snow metamorphosis, a better understanding of snowpack variability, and the ability to interpret wind-loading patterns and the consequences this has for avalanche risk.
Those familiar with test scores from stability tests rated the factor as moderately relevant but not very precise to assess. Most stability tests are time-consuming and require knowledge in interpreting the results (Techel et al., 2020).
Inclination (steepness) was rated as relevant. There are available tools and simple methods to assess inclination. However, actively using inclination measurements for avalanche risk assessment requires training and experience. Our results confirmed this by more precise assessments, the more avalanche education.
Relevance of avalanche terrain and non-avalanche terrain was high. The more avalanche education, the easier it was to distinguish avalanche terrain from non-avalanche terrain. In addition to release areas (terrain steeper than 30 degrees), runout zones must be recognized to assess this factor. High-resolution slope (inclination) maps in combination with computer-modeled runout zones (and possibly also release areas) available on smartphone apps simplify the assessment process of slope angle and avalanche terrain. NVEs smartphone app Varsom Regobs provides maps of slope angles above 30 degrees and avalanche runout zones across Norway and Svalbard (Larsen et al., 2020).
Forest density received the lowest relevance rating. The disparity in the results may reflect that forest density is really a twofold factor. On one hand, forest could be a terrain trap that will increase the consequence of an avalanche. On the other hand, forest could have a positive, stabilizing effect on the snow cover, which will reduce the possibility of triggering an avalanche. How one interprets the question will affect the answer.
Terrain traps increase the consequence for those getting caught in an avalanche and our respondents agreed upon the high relevance of this factor. The skill to identify terrain traps increased markedly with avalanche education.
Assessment of safe spots improved with avalanche education. This is a risk reduction measure that ensures safety in case of an avalanche release triggered by someone else in the group. Expedient use of this factor presupposes a high degree of understanding and reading terrain.
Implications for avalanche forecasting
Avalanche forecasts play an important role, both as a source for learning and as a source for information on the current avalanche situation, thus serving as a starting point for the avalanche risk assessment. Ideally, there should be a balance between the avalanche conditions, the groups’ competence, and the terrain selection. For example, if a group of backcountry skiers with little competence wants to go skiing at forecasted danger level 3-considerable and a problem of wind-drifted snow, they should choose low-angle terrain and avoid runout zones to reduce the risk of getting caught in an avalanche. In comparison, a group with high competence has more leeway in their terrain selection based on the information from the forecast and their ability to do local assessments. In a study of avalanche risk communication, St. Clair (2019) identified five types of avalanche bulletin users that differ widely in how they use avalanche forecast information and argues to facilitate user advancement to enable a more complete risk management strategy. She points at the untapped potential for an avalanche forecast to facilitate learning and improve user competence, viewing it as an educational tool “to improve users’ ability to conceptualize and manage the risk” (St. Clair, 2019, p. 68). In this study we tested the understanding of some of the avalanche forecast elements that could benefit from this view of the forecast as an educational tool.
For many amateur recreationalists, the avalanche forecast provides the most critical information for deciding to ski a slope (Furman et al., 2010; Marengo et al., 2017). Forecasts follow the information pyramid structure, where the avalanche danger level is presented at the top and regarded the most important forecast element. The danger level is a description of the overall complexity and severity of the avalanche situation in a region. The risk more than quadruples from one danger level to the next from level 1 to 3, not just a doubling as previously calculated (Winkler et al., 2021). These findings clearly show that understanding the danger level is crucial for risk management and that warning services must develop methods to make this concept comprehensible to the user. The weak layer characteristics (its distribution, depth, and type) are important to where and how easy it is for a skier to release a dry slab avalanche. Although it is generally considered difficult to precisely assess the weak layer distribution, it is an important task for avalanche warnings services and their professional observers to discover, monitor and communicate the existence and properties of such layers. As these factors are difficult to precisely assess in the field even for experts, the uncertainty and required caution need to be communicated in a clear and transparent manner by the warning services.
A commonly used phrase in avalanche education is “When snow is the problem, the terrain is the solution”. Forecasting services should provide relevant terrain information, like release and runout areas, or avalanche terrain classification, inclination, terrain traps, safe spots/areas, and forest density, making it easier for the users to balance group composition, avalanche conditions and terrain selection. Some of these functions already exist in the Varsom Regobs app.
Forecast elements, such as avalanche problems, demand a conceptual understanding and avalanche knowledge. This should not discourage the forecasting services from presenting these elements in a more appealing and easier understandable way by e.g., using illustrative pictures and informative video-clips. Several studies have documented a positive reception to these initiatives (Diegel & Tremper, 2012; Nariz et al., 2018). We recommend that avalanche warning services have close ties to educational institutions to foster comprehension and correct use of the forecast, or even integrate educational resources in their forecasting service.
Publishing real-time field observations, not only the regional avalanche warning, can improve risk management. The NAWS provides observations in real-time, such as signs of instability, distribution and depth of weak layers, and avalanche size. This increases transparency and allows learning about the uncertainty in risk assessment.
Benefits of avalanche education
Avalanche education increased the self-rated ability to assess the factors, an ability crucial for the quality of decisions in avalanche terrain. To have a positive accident prevention effect, we believe training must lead to both the development of specific skills and the ability to assess one’s own skills (Norman et al., 2019). Avalanche decision-making involves certain practical skills (i.e., measuring slope angle, interpreting snow profile information and stability tests), the ability to detect and monitor cues, and to seek relevant information. Some skills can be acquired through reading or personal experience. However, some skills are hard to learn (i.e., snowpack analysis) and require instruction. An experiential learning style appears to be the most suitable approach given the complexity of the subject and diverse skill requirements (McCarthy, 2010). Still, as we have pointed out, it also involves several challenges. Avalanche decision-making takes place in a wicked learning environment where natures’ feedback on assessment and decisions can be lacking or misleading (Hogarth et al., 2015; Stewart-Patterson, 2014). Hence, the competence of the instructor and her ability to facilitate an optimal learning environment and progression becomes crucial. Sometimes the instructors’ interpretation of a situation is necessary to reduce the multidimensionality and complexity of the experiences and make it available as knowledge to the student (Hertwig et al., 2018). This is especially important for beginners. In what can be referred to as deliberate guided practice, the instructor’s competence and understanding is the link between the student and learning (Magnussen, 2012). Done well, this provides a learning environment perfect for learning skills such as pattern recognition, detecting and monitoring cues, situational adaption and applying a decision-making structure. The instructor is also responsible for making the students aware of any experience if the student does not understand or notice it herself (Hertwig et al., 2018). One of the greatest strengths of experiential learning over descriptive learning is the closeness to the events and the action, providing a greater learning effect (Beames & Brown, 2016). The students get direct empirical knowledge from the experience, while descriptive learning must go through several processes where the experience is interpreted before it transforms into knowledge with the student. The more transparent this interpretation the more there is to be gained for the learners. Due to the dynamic environment, some of the learning taking place in the field is not predictable. This can make intentional learning difficult, but is positive in the sense that the student takes part in “discovering” the learning, often referred to as situational learning (Hallandvik & Høyem, 2019; Hofmann et al., 2018; Magnussen, 2012). This means that the situations one encounters determine the learning outcome of a given trip. For avalanche education, one will get real and contextual situations from this form of learning, but it will be challenging to achieve the intended learning for an avalanche course without sufficient time.
Repetition is what gives us the ability of recognizing patterns and contributes to our understanding (Faarlund, 2015; Ronglan, 2008). In avalanche education, repetition is time-consuming and sometimes impossible as conditions are dynamic and change over space and time. The conditions necessary for exemplifying or training a particular situation or skill might be absent or too dangerous. Nevertheless, repetition of experience is something to strive for because one can acquire skills that are difficult to acquire through description (Hertwig et al., 2018). In addition to the traditional field-based training, avalanche education should include video-based learning and interactive online-based learning tools like White risk (Harvey et al., 2013) or Know before you go (Gordon et al., 2016). This would amplify or prepare for the field-based training and may compensate for situations when factors are not present or too dangerous to approach (Kuiken & Twisk, 2001). However, video-based learning does not replace field-based learning. Crucially, instructors must provide adequate feedback and compare the students’ rationale with the rationale provided by the instructor or expert (Klein & Borders, 2016). Balent et al. (2018) reported that students following recreational avalanche education courses found activities that incorporated feedback from the instructor to be the most valuable.
Table 4 shows the necessary learning objectives (column 2), acquired skills (column 3) that should be incorporated in any formal avalanche training, relevance (column 4), at which stage of an outing the factor can be used (column 5–7), the effect of training on understanding avalanche risk (column 8), and how easy it is to become expedient (column 9). The latter is our recommendation based on weighting the ease of interpretation, level of uncertainty associated and the scope of learning tasks. We also recommend which factors are more suitable for video education (column 10), considering their degree of visual observability and conceptual complexity. The following factors are high in relevance and can be effectively improved by training: signs of instability, slope inclination, stopping at safe points, distribution and depth of weak layer, and avalanche size. Signs of instability and most terrain factors are relatively easy to learn, while stopping at safe points and avalanche terrain is more advanced and complex. The danger level is easy to learn, but also transforms complex snow and avalanche information into one single item and thus complex to understand. We identified six of the factors as prime candidates for educational videos: avalanche size, signs of instability, avalanche terrain, inclination, terrain traps, and safe stops.
Limitations
Our study did not use all 53 avalanche risk factors, as we focused on the role of avalanche education for key avalanche risk factors. Respondents were panelists and attendees at three different avalanche seminars, and although separate environments may influence results, we achieved samples with high natural variability in terms of avalanche education, experience and geography. The explained variance was low, suggesting that other predictors than avalanche education and experience contribute to the precision and relevance ratings. There might be differences in self-reported and actual in-field use, addressable in future research.
Conclusion
In high-stake low probability environment like snow-covered mountains, avalanche risk assessment requires both knowing the relevant factors and being able to assess those factors with sufficient precision. By studying the role of avalanche education on assessing and judging avalanche risk factors among amateur backcountry recreationalists, we found a similar judgement of which factors are relevant and predictive of avalanches as we previously found among experts. The ability to assess the factors with enough precision often depended, and even improved with avalanche education. Amateur recreationalists viewed avalanche risk assessment as learnable. This supports the view that analytical, knowledge-based decision-making should not be reserved for experts but should be taught to beginners as well. We point at pedagogical opportunities and challenges that must be considered for an improvement of avalanche forecasting, avalanche education and in the development of decision-making frameworks. We acknowledge that there always will be residual risk and uncertainty, making it impossible to guarantee absolute safety.
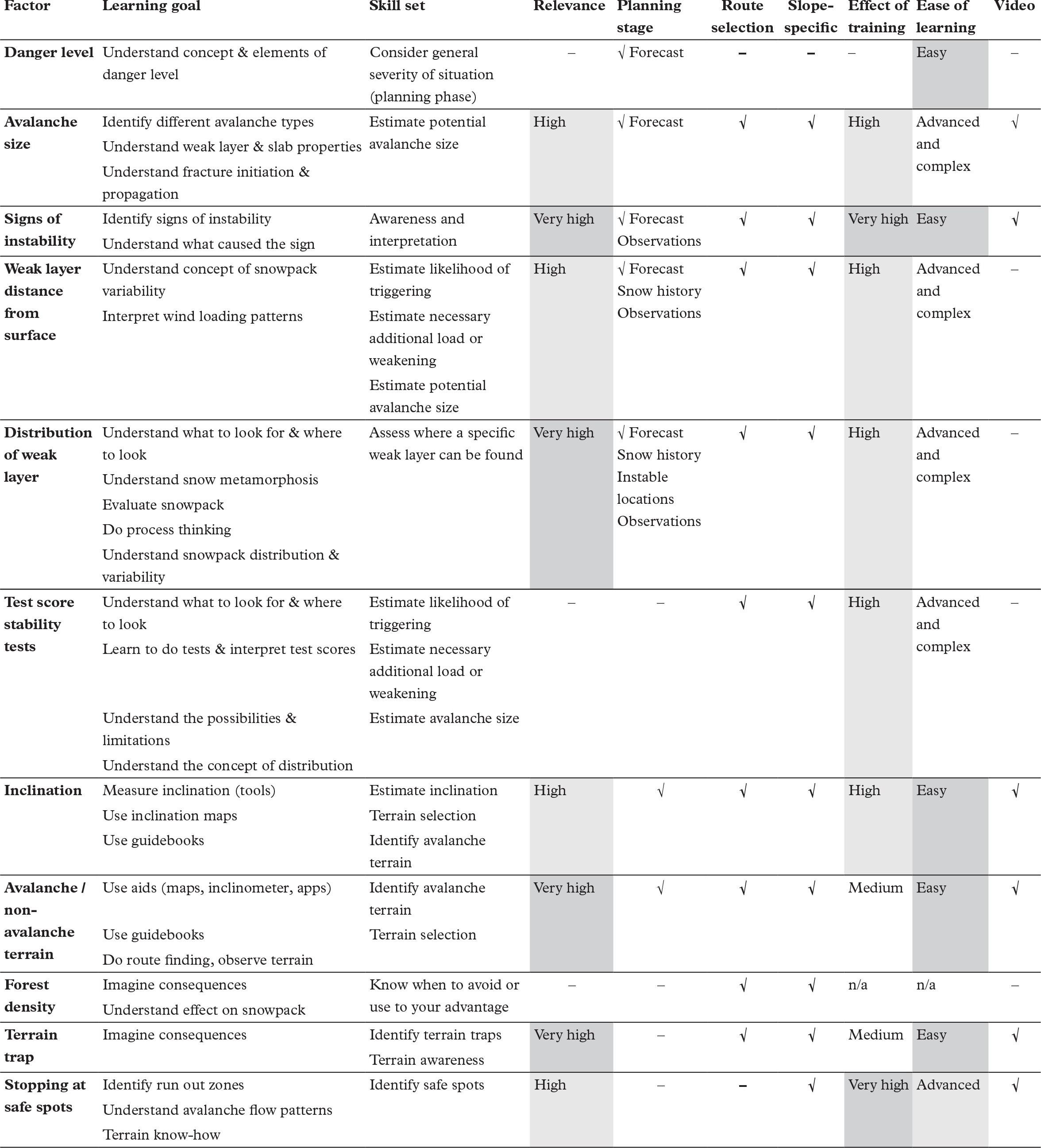
Acknowledgements
The publication charges for this article have been funded by a grant from the publication fund of UiT – The Arctic University of Norway.
References
- Atkins, D., & McCammon, I. (2004). Differences between avalanche experts and novices. In Proceedings of the 2004 International Snow Science Workshop, Jackson Hole, Wyoming, USA (pp. 452–461). https://arc.lib.montana.edu/snow-science/item/1117
- Balent, C., Johnson, J., Hendrikx, J., & Shanahan, E. (2018). Theory and practice in introductory avalanche education. In Proceedings of the 2018 International Snow Science Workshop, Innsbruck, Austria (pp. 1462–1466). https://arc.lib.montana.edu/snow-science/item/2798
- Beames, S., & Brown, M. (2016). Adventurous learning. A pedogogy for a changing world. Routledge.
- Bonini, N., Pighin, S., Rettore, E., Savadori, L., Schena, F., Tonini, S., & Tosi, P. (2019). Overconfident people are more exposed to “black swan” events: A case study of avalanche risk. Empirical Economics, 57(4), 1443–1467. https://doi.org/10.1007/s00181-018-1489-5
- Diegel, J. D., & Tremper, B. (2012). @Utahavalanche: Was social media invented for avalanche forecasting? In Proceedings of the 2012 International Snow Science Workshop, Anchorage, AK, USA (pp. 387–391). https://arc.lib.montana.edu/snow-science/item/1705
- Dunlap, A. S., & Stephens, D. W. (2016). Reliability, uncertainty, and costs in the evolution of animal learning. Current Opinion in Behavioral Sciences, 12, 73–79. https://doi.org/10.1016/j.cobeha.2016.09.010
- EAWS. (2019). Summary 20th EAWS General Assembly 2019 Oslo, Norway. Retrieved 24.08 from https://www.avalanches.org/wp-content/uploads/2019/07/general-assembly-oslo_minutes_EAWS.pdf
- Elwin, E. (2013). Living and learning: Reproducing beliefs in selective experience. Journal of Behavioral Decision Making, 26(4), 327–337. https://doi.org/10.1002/bdm.1770
- Engeset, R., Pfuhl, G., Landrø, M., Mannberg, A., & Hetland, A. (2018). Communicating public avalanche warnings – what works? Natural Hazards and Earth System Sciences, 18(9), 2537–2559. https://doi.org/10.5194/nhess-18-2537-2018
- Ericsson, K. A., Krampe, R. T., & Tesch-Römer, C. (1993). The role of deliberate practice in the acquisition of expert performance. Psychological Review, 100(3), 363–406. https://doi.org/10.1037/0033-295X.100.3.363
- Funder, D. C., & Ozer, D. J. (2019). Evaluating effect size in psychological research: Sense and nonsense. Advances in Methods and Practices in Psychological Science, 2(2), 156–168. https://doi.org/10.1177/2515245919847202
- Furman, N., Shooter, W., & Schumann, S. (2010). The roles of heuristics, avalanche forecast, and risk propensity in the decision making of backcountry skiers. Leisure Sciences, 32(5), 453–469. https://doi.org/10.1080/01490400.2010.510967
- Faarlund, N. (2015). Friluftsliv. En dannelsesreise. Ljå Forlag.
- Gordon, C., Diegel, P., Meisenheimer, T., Lazar, B., Green, E., Clayton, M., Carlson, A., Tremper, B., Staples, M., Birkeland, K., & Bennett, T. (2016). Know before you go revision: An introductory tool for influencing backcountry behavior. In Proceedings of the 2016 International Snow Science Workshop, Breckenridge, Colorado, USA (pp. 290–293). https://arc.lib.montana.edu/snow-science/item/2281
- Hallandvik, L., & Høyem, J. (2019). Friluftslivspedagogikk. Cappelen Damm Akademisk.
- Harvey, S., Aegerter, S., & Landolt, D. (2013). White Risk 2.0 – A new web-based platform for avalanche education. In Proceedings of the 2013 International Snow Science Workshop, Grenoble, France (pp. 507–510). https://arc.lib.montana.edu/snow-science/item/1990
- Harvey, S., Rhyner, H., Dürr, L., & Henny, H., M. (2018). To go or not to go: Decision making at individual slope. In Proceedings of the 2018 International Snow Science Workshop, Innsbruck, Austria (pp. 1346–1350). https://arc.lib.montana.edu/snow-science/item/2771
- Harvey, S., Rhyner, H., Dürr, L., Schweizer, J., & Henny, H. M. (2018). Caution avalanches! The Snow Sport Avalanche Accident Prevention core training team. WSL Institute for Snow and Avalanche Research SLF.
- Hertwig, R., Hogarth, R. M., & Lejarraga, T. (2018). Experience and description: Exploring two paths to knowledge. Current Directions in Psychological Science, 27(2), 123–128. https://doi.org/10.1177/0963721417740645
- Hofmann, A., R, Rolland, C., Rafoss, K., & Zoglowek, H. (2018). Norwegian Friluftsliv. A way of living and learning in nature. Waxmann Verlag GmbH.
- Hogarth, R. M., Lejarraga, T., & Soyer, E. (2015). The two settings of kind and wicked learning environments. Current Directions in Psychological Science, 24(5), 379–385. https://doi.org/10.1177/0963721415591878
- Klein, G., & Borders, J. (2016). The ShadowBox Approach to cognitive skills training. Journal of Cognitive Engineering and Decision Making, 10(3), 268–280. https://doi.org/10.1177/1555343416636515
- Kronthaler, G. (2019). 20 Jahre „Systematische Schneedeckendiagnose“. Bergundsteigen, 106, 17–27.
- Kuiken, M., & Twisk, D. (2001). Safe driving and the training of calibration. SWOV Institute for Road Safety Research, The Netherlands.
- Landrø, M., Hetland, A., Engeset, R. V., & Pfuhl, G. (2020). Avalanche decision-making frameworks: Factors and methods used by experts. Cold Regions Science and Technology, 170, 1–11. https://doi.org/10.1016/j.coldregions.2019.102897
- Landrø, M., Pfuhl, G., Engeset, R., Jackson, M., & Hetland, A. (2020). Avalanche decision-making frameworks: Classification and description of underlying factors. Cold Regions Science and Technology, 169, 1–14. https://doi.org/10.1016/j.coldregions.2019.102903
- Larsen, H., Hendrikx, J., Slatten, M., & Engeset, R. (2020). Developing nationwide avalanche terrain maps for Norway. Natural Hazards, 103(3), 2829–2847. https://doi.org/10.1007/s11069-020-04104-7
- Maekelae, M. J., & Pfuhl, G. (2019). Deliberate reasoning is not affected by language. PLOS ONE, 14(1), 1–13. https://doi.org/10.1371/journal.pone.0211428
- Magnussen, L. I. (2012). Læring i Friluftsliv. Om padlefellesskap i havgapet [PhD thesis]. Norges Idrettshøgskole.
- Marengo, D., Monaci, M. G., & Miceli, R. (2017). Winter recreationists’ self-reported likelihood of skiing backcountry slopes: Investigating the role of situational factors, personal experiences with avalanches and sensation-seeking. Journal of Environmental Psychology, 49, 78–85. https://doi.org/10.1016/j.jenvp.2016.12.005
- McCammon, I., Haegeli, P. (2004). Comparing avalanche decision frameworks using accident data from the United States. In Proceedings of the 2004 International Snow Science Workshop, Jackson, WY, USA (pp. 1–11). https://arc.lib.montana.edu/snow-science/item/1126
- McClung, D., & Schaerer, P. (2006). The avalanche handbook (3. ed.). The Mountaineers Books.
- Morey, R. D., Rouder, J. N., Jamil, T., Urbanek, S., Forner, K., & Ly, A. (2018). BayesFactor: Computation of Bayes factors for common designs (Version 0.9.12-4.1) [Computer software]. Comprehensive R Archive Network. https://CRAN.R-project.org/package=BayesFactor
- Nariz, P., Ruetz, L., & Kriz, K. (2018). How to assess and communicate persistent weak layers: A forecaster's perspective. In Proceedings of the 2018 International Snow Science Workshop, Innsbruck, Austria (pp. 1553–1558). https://arc.lib.montana.edu/snow-science/item/2818
- Norman, E., Pfuhl, G., Sæle, R. G., Svartdal, F., Låg, T., & Dahl, T. I. (2019). Metacognition in psychology. Review of General Psychology, 23(4), 403–424. https://doi.org/10.1177/1089268019883821
- Pfuhl, G., Tjelmeland, H., & Biegler, R. (2011). Precision and reliability in animal navigation. Bulletin of Mathematical Biology, 73(5), 951–977. https://doi.org/10.1007/s11538-010-9547-y
- Reuter, B., Semmel, C., Mallon, A., & Schweizer, J. (2021). Risikomanagement da capo. Bergundsteigen, 113, 48–55.
- Ronglan, L. T. (2008). Lagspill, læring og ledelse. Akilles.
- Schweizer, J., & Lütschg, M. (2000). Measurements of human-triggered avalanches from the Swiss alps. In Proceedings of the 2000 International Snow Science Workshop, Big Sky, Montana, USA (pp. 1–8). https://arc.lib.montana.edu/snow-science/item/729
- St. Clair, A. (2019). Exploring the effectiveness of avalanche risk communication: A qualitative study of avalanche bulletin use among backcountry recreationists [Master thesis]. Simon Fraser University.
- Statham, G., Haegeli, P., Greene, E., Birkeland, K., Israelson, C., Tremper, B., Stethem, C., McMahon, B., White, B., & Kelly, J. (2018). A conceptual model of avalanche hazard. Natural Hazards, 90(2), 663–691. https://doi.org/10.1007/s11069-017-3070-5
- Stephens, D. W. (1991). Change, regularity, and value in the evolution of animal learning. Behavioral Ecology, 2(1), 77–89. https://doi.org/10.1093/beheco/2.1.77
- Stewart-Patterson, I. (2014). The development of ski guide expertise. In Proceedings of the 2014 International Snow Science Workshop, Banff, Canada (pp. 330–337). https://arc.lib.montana.edu/snow-science/item/2074
- Stewart, T. R., Mumpower, J. L., & James Holzworth, R. (2012). Learning to make selection and detection decisions: The roles of base rate and feedback. Journal of Behavioral Decision Making, 25(5), 522–533. https://doi.org/10.1002/bdm.755
- Techel, F., Winkler, K., Walcher, M., van Herwijnen, A., & Schweizer, J. (2020). On snow stability interpretation of extended column test results. Natural Hazards and Earth System Sciences, 20(7), 1941–1953. https://doi.org/10.5194/nhess-20-1941-2020
- Ulkumen, G., Fox, C. R., & Malle, B. F. (2016). Two dimensions of subjective uncertainty: Clues from natural language. Journal of Experimental Psychology: General, 145(10), 1280–1297. https://doi.org/10.1037/xge0000202
- Wetzels, R., & Wagenmakers, E.-J. (2012). A default Bayesian hypothesis test for correlations and partial correlations. Psychonomic Bulletin & Review, 19(6), 1057–1064. https://doi.org/10.3758/s13423-012-0295-x
- Winkler, K., Schmudlach, G., Degraeuwe, B., & Techel, F. (2021). On the correlation between the forecast avalanche danger and avalanche risk taken by backcountry skiers in Switzerland. Cold Regions Science and Technology, 188, 1–17. https://doi.org/10.1016/j.coldregions.2021.103299